Surrogate models are transforming how you optimize electrical machine design by simplifying complex analyses and balancing multiple objectives like efficiency and cost. They leverage advanced techniques, such as machine learning, to enhance accuracy while reducing the need for costly experiments. However, challenges like data requirements and model efficiency persist. You'll discover innovative solutions and strategies in this evolving field that can further improve your designs—stay tuned to uncover more insights on maximizing your optimization efforts.
Key Takeaways
- Surrogate models effectively simplify complex electrical machine analysis, enhancing design optimization speed and efficiency while minimizing reliance on expensive physical experiments.
- Integrating machine learning with surrogate models improves prediction accuracy, especially in nonlinear scenarios, using hybrid approaches that combine data-driven and physical models.
- Multi-objective optimization techniques, such as WTSM and Kriging, facilitate balancing competing design goals, optimizing efficiency while reducing resource expenditure.
- Challenges in surrogate modeling include the need for large datasets and the difficulty of balancing accuracy with computational efficiency, which ongoing research aims to address.
- Industry adoption of surrogate models is increasing, showcasing practical benefits and fostering innovation, with future advancements expected to enhance modeling capabilities further.
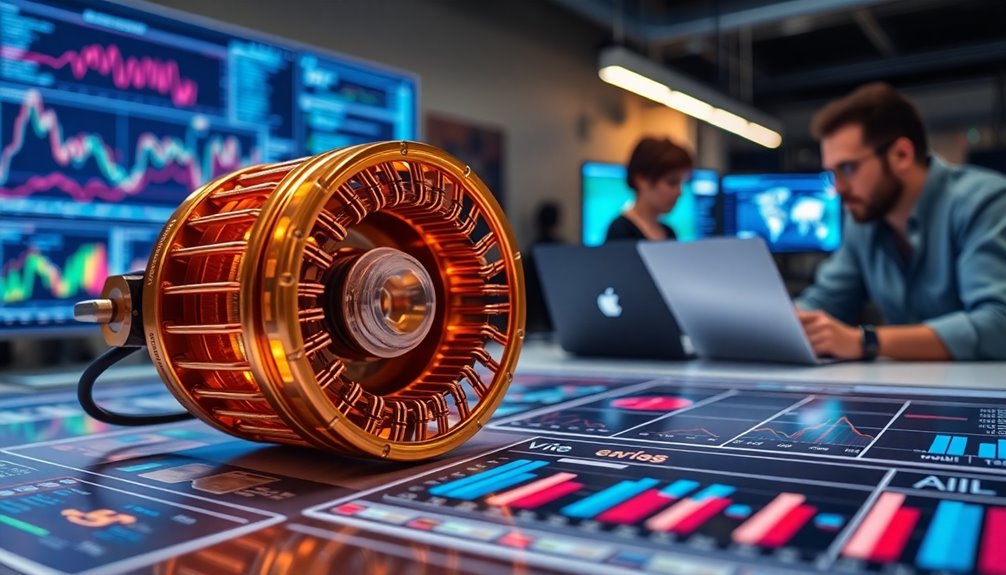
In electrical machine design, surrogate models play a crucial role in simplifying the analysis of complex systems. These models approximate the behavior of electrical machines, reducing your reliance on costly physical experiments and intricate mathematical formulations. You'll find that common techniques, like neural networks and gradient boosted decision trees, help streamline this process, making design optimization not only faster but also more efficient.
Surrogate models revolutionize electrical machine design, simplifying analysis and boosting efficiency through advanced techniques like neural networks.
By integrating machine learning, especially neural networks, surrogate models become even more powerful. You can use data-driven approaches that require less computational power than traditional finite element analysis (FEA), although they may still need large datasets for high accuracy. This is where hybrid models shine, combining data-driven techniques with physical models to enhance prediction accuracy, particularly in nonlinear scenarios.
When you apply multi-objective optimization techniques, surrogate-assisted approaches allow you to balance competing design goals, such as efficiency and cost. The computational efficiency you gain from surrogate models significantly reduces the time and resources spent on traditional optimization methods. Techniques like Waveform-Targeted Surrogate Modeling (WTSM) and the Kriging model, often combined with Latin Hypercube Sampling, further improve the design process by refining model construction. Additionally, surrogate-assisted modeling has shown to enhance accuracy and robustness in design optimization, making it a vital tool in modern engineering.
However, challenges remain. You'll need to balance model accuracy with computational efficiency and often require large datasets. Fortunately, techniques like transfer learning can help mitigate data demands. Embracing a hybrid approach can lead to better predictions, particularly for complex phenomena. As research progresses, expect to see advances that enhance surrogate model accuracy while reducing data requirements.
Industries are increasingly adopting these surrogate models for efficient design optimization, showcasing their practical benefits. By leveraging surrogate models in your electrical machine design projects, you not only optimize performance but also pave the way for innovative solutions in a competitive landscape. The future is bright for those willing to explore these advanced modeling techniques.
Frequently Asked Questions
What Are the Main Advantages of Using Surrogate Models in Design Optimization?
Surrogate models offer you several advantages in design optimization. They significantly reduce computational costs and speed up the optimization process by allowing faster iterations and minimizing sample sizes.
You'll find that they enhance accuracy and reliability, providing consistent approximations and detailed insights into design-performance relationships.
Additionally, their versatility lets you integrate them across various disciplines, helping you balance competing objectives effectively while making informed decisions quickly.
How Do Surrogate Models Handle Uncertainty in Electrical Machine Design?
Imagine navigating a stormy sea without a compass. Surrogate models act as your guiding light, helping you handle uncertainty in electrical machine design.
They approximate complex models, reducing computational costs while quantifying uncertainties from design variations and material properties.
What Software Tools Are Commonly Used for Surrogate Modeling?
When you're diving into surrogate modeling, several software tools stand out. You might consider using Kriging for spatial modeling or MATLAB's SUMO for various scientific tasks.
Python SMT is great for its derivative features, while Julia's SciML/Surrogates.jl offers robust tools for creating and optimizing models.
Don't overlook Scikit-learn, which provides handy methods like kriging and linear approaches for constructing surrogates. Each tool has unique strengths to enhance your modeling efforts.
Can Surrogate Models Be Applied to Other Engineering Fields Besides Electrical Machines?
Imagine a skilled artist using a brush to create stunning landscapes. Just like that, surrogate models paint solutions across various engineering fields.
Yes, you can definitely apply these models beyond electrical machines. They're carving pathways in aerospace for wing optimization, shaping civil structures under loads, and refining processes in energy and manufacturing.
Each application showcases their versatility, transforming complex challenges into manageable tasks with remarkable efficiency and precision.
How Do You Validate the Accuracy of a Surrogate Model?
To validate the accuracy of a surrogate model, you should use techniques like cross-validation and leave-one-out error estimation.
These methods help you assess predictive performance and avoid overfitting. Incorporating design of experiments can optimize sample selection for better results.
Additionally, consider applying Bayesian optimization to fine-tune your model.
Conclusion
So, you thought electrical machine design was all about fancy equations and endless simulations? Think again! Surrogate models are the unsung heroes, turning your complex multi-objective nightmares into manageable dreams. But let's be real: while they promise efficiency, they also throw a wrench in the works with their own set of challenges. It's a wild ride, balancing speed and accuracy, but hey, who doesn't love a good challenge? After all, nothing says "fun" like optimizing chaos!